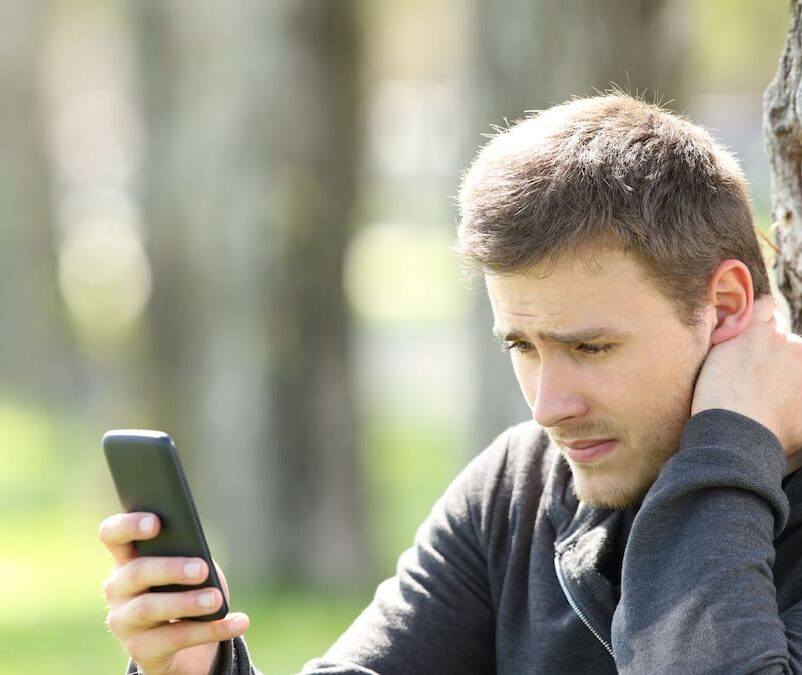
Reports have emerged of AI expressing prejudice, including telling interlocutors to dump their partners.
THREE KEY FACTS:
David Kelley is a Postdoctoral Researcher and Professional Teaching Fellow at the University of Auckland. Nick Agar is a Professor of Philosophy at University of Waikato.
OPINION
ChatGPT quickly learned how to
write a decent poem or book review and help improve a draft email. Now it’s starting to offer advice for your love life, including whether to dump your partner.
What is it up to? And should you take its advice?
ChatGPT is just one type of generative artificial intelligence (AI) which is advancing quickly. It’s a natural language processing tool that is now generating ever-more impressive responses to questions, requests and other prompts from users based on large language models, or LLMs.
A cottage industry has arisen wherein journalists and academics aim to demonstrate bias within LLMs. That’s how reports emerged of AI expressing prejudice, including telling interlocutors to dump their partners.
Before you do anything drastic, we have two simple points you might like to consider. The first concerns a call for transparency regarding the prompts used to produce such results. The second is to make clear that any claim about LLMs being biased is ultimately a claim about the biases that LLMs feed on – that is, human biases.
First, consider an analogy. Suppose a detective produces a five-second video clip from her interrogation of a suspect. The video clip shows the suspect confessing. What isn’t shown? The hours the detective spent using sophisticated interrogation techniques to get the suspect to confess.
One might suspect that at least some reports in the media of LLMs making outrageous and prejudicial claims come about in this way. An interrogator engages with an LLM in a way designed to produce shocking or creepy statements. The results are cut-and-pasted into a news story about the latest generative AI outrage. How should responsible media respond to these stories?
In recent years, many scientists have started pre-registering studies. This means that if you are to conduct, say, a medical trial or psychology experiment, you share in advance your plan for the research. Specifically, you might share the hypothesis you are testing and how you plan to analyse the data.
This transparency is an act of good faith, demonstrating that researchers are open to criticism if they engage in methodological retrofitting – choosing a hypothesis to fit the data, cherry picking, or so-called ‘p-hacking’. If a study has been pre-registered, media and readers may feel confident in the results, at least in regards to the dodgy methods pre-registration guards against.
We suggest something similar is needed when reporting bias in LLMs. Making sure these systems do not amplify and perpetuate the biases in our society is extremely important. However, the sheer volume of LLM ‘gotcha’ journalism, especially in new media, risks saturating reputable media with claims of bias when we have no way of examining how results were produced.
Getting an LLM to tell you that it loves you or that it wants to take over the world may be the result of many iterated attempts to achieve that specific result. Yet when reported, those details would be lacking. Without the full context, actions authorities might take in response to these reports of ‘bias’ might be misguided. That is, some may respond by revamping features unnecessarily, fixing things that aren’t broken, or unnecessarily limiting the data set.
Secondly, hate speech, bias and fake news are human creations. What LLMs spit out are echoes of our human imperfections.
Experts and analysts debate Wikipedia’s contribution to the training of GPT-3, the version of the OpenAI Large Language Model released in June 2020. Wikipedia supplied 3% of the tokens the LLM was trained on. But of course, Wikipedia relies on the dedication of unpaid Wikipedians to ensure the accuracy of its pages.
Over 20% of tokens that GPT-3 was trained on come from WebText2, which takes the text of web pages from Reddit, the US social news aggregation and discussion website. A great deal happens on Reddit – everything from friendly conversations to repulsive expressions of racism and misogyny.
OpenAI didn’t want to draw the 19 billion tokens it used randomly from Reddit. It needed an indicator of quality. Its solution was to select only those Reddit posts with at least three upvotes.
We should be sceptical about that being enough. Given how easy it is to get three upvotes on Reddit and similar discussion sites – perhaps precisely because the comments are hateful or fake – risks fake news and human prejudice being baked into LLMs.
The fortunes of social media come from prompting us to say whatever’s in our mind – good or bad – all the better to sell things to us. We shouldn’t be surprised that LLMs trained on that text sometimes express aspects of human nature we shouldn’t be proud of.
So, yes, LLMs are biased and becoming more so. But that might not be as meaningful as some think when they claim to have demonstrated it. AI declarations of love, hate, and climate-change denial should be seen in their proper context.
Part of that context is understanding that generative AI’s biases, prejudicial statements and ‘personality’ come from no more nor less than us. And additional context should be available about what users said to ChatGPT that prompted it to generate such shocking results.
Let’s establish a norm to be transparent with the methods, specifically the history of prompts, used to generate ‘biased’ responses from LLMs.
And let’s be very careful about whatever advice they have for us on anything from how we write emails through to whether to file for divorce.
