Nvidia has a broad effort to use Artificial Intelligence for Quantum Computing.
AI can play a role in everything from designing qubits, preparing efficient quantum algorithms, controlling and calibrating the device, correcting errors in realtime, and interpreting the output from QC. Most importantly, each aspect of QC needs to scale, and AI might be the only tool with the ability to both solve these problems effectively and do so efficiently at scale. AI has only begun to benefit QC and it is likely that AI will play an increasingly critical role into the realization of useful QC applications and FTQC.
Artificial intelligence (AI) advancements over the past few years have had an unprecedented and revolutionary impact across everyday application areas. Its significance also extends to technical challenges within science and engineering, including the nascent field of quantum computing (QC). The counterintuitive nature and high-dimensional mathematics of QC make it a prime candidate for AI’s data-driven learning capabilities, and in fact, many of QC’s biggest scaling challenges may ultimately rest on developments in AI. However, bringing leading techniques from AI to QC requires drawing on disparate expertise from arguably two of the most advanced and esoteric areas of computer science. Here we aim to encourage this cross-pollination by reviewing how state-of-the-art AI techniques are already advancing challenges across the hardware and software stack needed to develop useful QC – from device design to applications. We then close by examining its future opportunities and obstacles in this space.
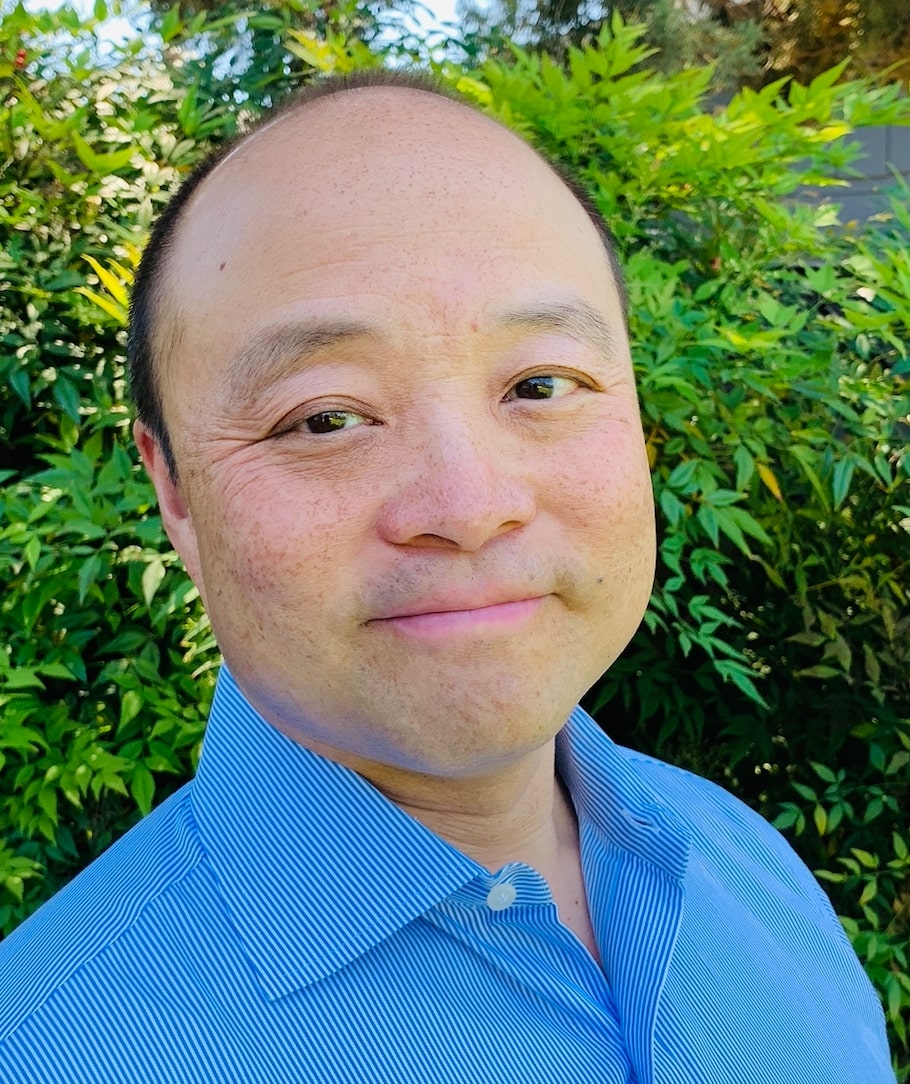
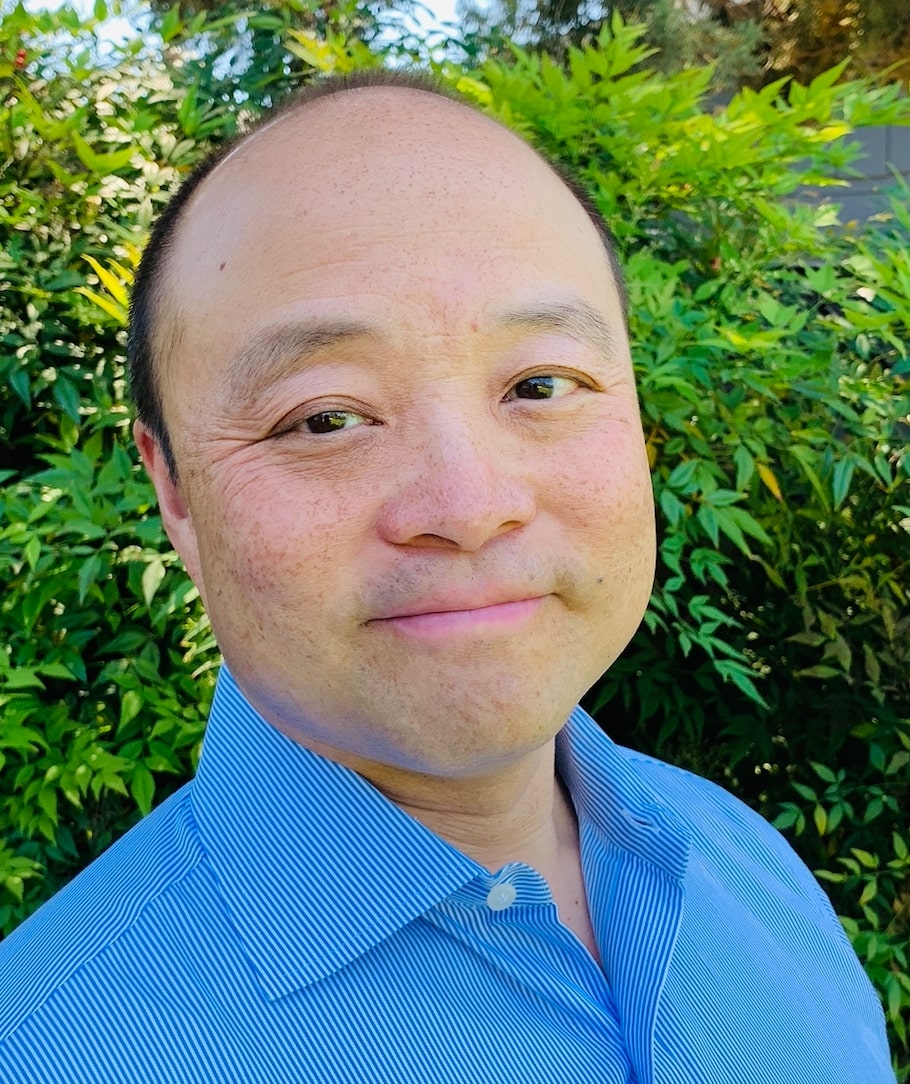
Brian Wang is a Futurist Thought Leader and a popular Science blogger with 1 million readers per month. His blog Nextbigfuture.com is ranked #1 Science News Blog. It covers many disruptive technology and trends including Space, Robotics, Artificial Intelligence, Medicine, Anti-aging Biotechnology, and Nanotechnology.
Known for identifying cutting edge technologies, he is currently a Co-Founder of a startup and fundraiser for high potential early-stage companies. He is the Head of Research for Allocations for deep technology investments and an Angel Investor at Space Angels.
A frequent speaker at corporations, he has been a TEDx speaker, a Singularity University speaker and guest at numerous interviews for radio and podcasts. He is open to public speaking and advising engagements.