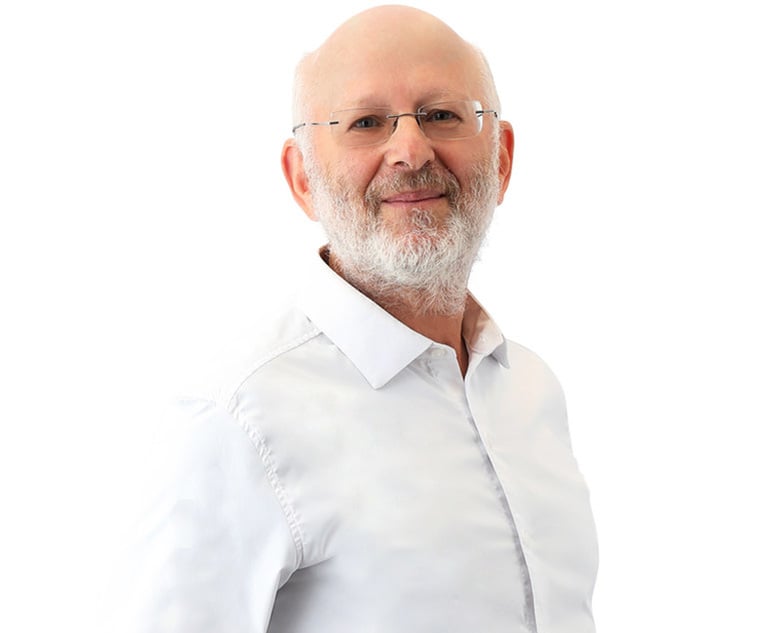
As the use of Artificial Intelligence (AI) increases, so does the likelihood of its role in both civil and criminal proceedings. While AI is often conceived of as a computer which can match or exceed a human’s performance in tasks requiring cognitive abilities, in fact it is just software. Software is generally admissible as evidence if it is relevant, material, and competent. However, AI differs from traditional software, perhaps requiring novel admissibility considerations.
More specifically, both traditional software and AI contain algorithms. Algorithms are procedures employed for solving a problem or performing a computation. Algorithms act as a step-by-step list of instructions specifying specific actions to be performed using either hardware or software-based routines. The fundamental difference with AI and traditional algorithms is that an AI can change its outputs based on new inputs, while a traditional algorithm will always generate the same output for a given input.
AI like traditional software may produce two types of evidence, namely computer records and computer generated evidence. Computer records do not require analysis or assumption by the programing, whereas computer generated evidence does. Computer records are generally print outs complied by a computer in a prescribed fashion from data. Computer generated evidence is computer output based on data and assumptions contained in a program.
The admissibility of both computer records and computer generated exhibits are the same as they are for traditional paper business records and traditional demonstrations, respectively. The fact that a computer is involved does not change the admissibility standards or procedures.
The identification and authentication of both paper business records and computer records are the same as any other writing (Federal Rules of Evidence 1001(1)(3). Both are no less hearsay than any other event they proport to prove. Consequently, they must qualify as to the rule against hearsay to be admissible. Admissibility, requires a proper foundation (See for example, United States v. Catabran 836 F 2nd 453 (1988)). More specifically, courts usually require the records was mase in the ordinary course of business, they are identified by a qualitied witness and the sources, method and timely of the preparation suggest the records are trustworthy.
Two types of computer generated evidence exist. The first is demonstrative and the second is experimental. Demonstrative evidence is normally static information such as a computer assisted design of a pipe after it is broken open. Experimental evidence is typically dynamic information, such as the output of a computer model resulting in a simulation of a pipe in the process of breaking.
Static depictions used in litigation are nearly always subject to the same admissibility rule, without regard to the role of a computer in their creation. The standard is simply does the representation accurately describe what it proports to illustrate. Such a representation does not require an expert witness.
Dynamic evidence (such as simulations) normally requires an expert to attest that the simulation for example, is derived from principles and procedures which generally accepted scientific standards (See generally, Frye v. United States 293 F 1013 (1938)). Additionally, admissibility requires some amount of experimental testing to confirm the simulation accords with reality.
Among the most serious concerns related to AI generated algorithms and their outputs is the lack of proper evaluation. AI are trained on data, and they learn to make algorithms which in turn make predictions by finding patterns in the data. However, if the training data is incomplete or biased, the AI may learn incorrect patterns. This can lead to the AI model making incorrect predictions, or hallucinating (See matters related to Roberto Mata v. Avanca Case 1:22-cv-01461-PKC such as https://www.documentcloud.org/documents/23826751-mata-v-avianca-airlines-affidavit-in-opposition-to-motion?responsive=1&title=1).
Admissibility of AI evidence is likely to face objections dues to the lack of rigorous testing because the AI algorithms that can have a significant impact on legal rights. Even when AI algorithm testing is performed, it is rarely independent, peer-reviewed, or sufficiently transparent to be properly assessed by those competent to do so. This shortcoming is likely to result in objections to AI evidence admissibility in accordance with the Frye Standard.
More specifically, the Frye Standard requires the admissibility of computer base evidence, such as AI evidence to be based on scientific methods that are sufficiently established and accepted. Since there are no standards for AI algorithms testing generally nor AI product testing specifically, it will be difficult to have an expert opinion that the AI evidence is admissible because it is “generally accepted” as reliable in the relevant scientific community.
Prior to the admissibility of AI evidence, courts should require transparency and explainability, both in terms of how the AI system works, as well as how it reached its decision (i.e., will a pipe rupture), classification (i.e., while pipes are eligible for a proper simulation), or prediction/conclusion (i.e., did a test pipe rupture as predicted?).
In short, AI evidence admissibility related to high-stakes decisions must provide explanations that reveal their inner workings and how the AI amends its algorithms such that AI algorithms are explainable. Evidence admissibility rules generally require evidence to be relevant, material, and competent. Competent evidence is understood to mean relevant evidence admissible in a particular action; that is relevant evidence not subject to the operation of any exclusionary rule (see People v Brewster, 100 A.D.2d 134 (1984)).
Evidence is “competent” if it complies with certain traditional notions of reliability (i.e. trustworthy). As applied to AI evidence, such evidence may be found trustworthy if it can explain the reason(s) for all outputs and show that the AI system’s process for generating the outputs is correct.
Another challenge for AI evidence admissibility is resilience. Resilience is the degree to which an AI resists both intentional and unintentional efforts to cause machine-learning models to fail.
Currently, AI which relies on Internet data for learning may develop malfunctioning algorithms due to spoofing. Often spoofing is the act of disguising the source of an Internet communication from an unknown source as being from a known, trusted source. Spoofing may first lead to erroneous AI machine learning, subsequently to faulty algorithms and finally untrustworthy AI evidence.
It is increasingly difficult to distinguish human generated Internet content from AI generated Internet content. Consequently, AI’s have been used to corrupt other AI’s. This practice known as adversarial AI uses AI to fool machine-learning models by supplying deceptive input(s). Adversarial AI can be used to modify the output of most AI technology.
While AI employs technology which may exceed human cognitive ability, never-the-less the rules of evidence do not disclose a separate evaluation standard. Thus, evidence gleaned from AI should be judged by the standard of direct witness testimony, expert witness testimony, or measurement using established technology. In sum, AI evidence is subject to the same rules of evidence as non-AI sources.
Consider Federal Rule of Evidence 401 which defines relevance. Rule 401 indicates that evidence is relevant if: (a) it has any tendency to make a fact more or less probable than it would be without the evidence; and (b) the fact is of consequence in determining the action.
Rule 401 is normally considered in conjunction with Rules 402 and 403. More specifically, Federal Rule of Evidence 402 discloses that the admissibility of evidence in federal court is normally based on relevance. Unless specifically prohibited, relevant evidence is admissible. Rule 403 limits Rule 402 by excluding relevant evidence if its probative value is outweighed by prejudice, confusion, or waste of time.
As related to the admissibility of AI evidence, Rule 403 has two important features. First, Rule 403 identifies the trial judge as the decision maker. Second, Rule 403 states that a judge cannot make the determinations unless the party offering the AI evidence is prepared to disclose underlying information. This would include the training data, as wells as the development and operation of the AI system sufficient to allow the opposing party to challenge it.
Thus, AI evidence for either civil or criminal trials, should not be permitted if the information underlying the AI is not available. Such information must be sufficient for the party against whom that evidence will be offered to determine the validity (including the accuracy of the Aid) and the reliability ( i.e., the AI algorithm correctly measures what it purports to measure).
As in the case of non-AI information, the trial judge should give the proponent of the AI evidence a choice. The proponent may either disclose the underlying evidence (perhaps under an appropriate protective order), or otherwise demonstrate its validity and reliability. If the proponent is unwilling to do so, the AI evidence should not be admissible.
Federal Rule of Evidence 901(a) requires AI evidence to be authenticated prior to consideration by the jury. Rule 901(b) discloses a variety of ways in which a party can achieve this objective. No special exception is made for AI evidence. A witness with knowledge of the AI will testify that the AI is what it is claimed to be (in accordance with Rule 901 (a)); and then that witness in accordance with Rule 901(b) will describing a process or system and showing that it produces an accurate result. Since AI programing is not common knowledge, it is expected that Rule 602 will apply requiring the authenticating witness to have personal knowledge of how the AI technology functions or be an expert.
Since AI usually requires both machine learning and generative elements, it is unlikely that a single witness will be sufficient for admissibility purposes. More specifically, AI machine normally requires one set of skills to teach a computer to understand certain data and perform certain tasks. Generative AI normally requires one set of skills to builds on that foundation and adds new capabilities that attempt to mimic human intelligence, creativity and autonomy.
Jonathan Bick is counsel at Brach Eichler in Roseland, and chairman of the firm’s patent, intellectual property, and information technology group. He is also an adjunct professor at Pace Law School and Rutgers Law School.