
Artificial Intelligence (AI) has emerged as a transformative technology, reshaping industries and driving innovations across a wide spectrum of applications. Behind every successful AI solution lies a vast amount of data, often referred to as the “new oil.” Data fuels AI models, enabling them to learn, improve, and make accurate predictions. However, the process of extracting meaningful insights from raw data and leveraging it for AI solutions is a complex and intricate journey.
The Relationship Between Data and AI
At its core, Artificial Intelligence thrives on data. Whether it’s predictive algorithms in financial markets, recommendation systems in e-commerce, or autonomous driving technologies, AI models rely heavily on data to function effectively. The performance of these models directly correlates with the quantity and quality of the data they are trained on. Without comprehensive and accurate data, even the most sophisticated AI systems can fail to deliver accurate results.
Data analysis bridges the gap between raw data and actionable insights. It involves cleaning, transforming, and interpreting data to discover patterns, relationships, and trends. AI solutions depend on this thorough analysis to learn from historical data, identify anomalies, and make informed decisions in real-time scenarios. Essentially, data analysis acts as the foundation upon which AI models are built and optimized.
Types of Data Used in AI
There are two main categories of data that AI systems utilize: structured and unstructured data.
Structured Data: This type of data is highly organized and easy to analyze. It fits neatly into rows and columns, making it ideal for relational databases. Examples include spreadsheets, customer records, and inventory lists. AI algorithms can quickly process structured data to make sense of it and provide useful insights.
Unstructured Data: Unlike structured data, unstructured data lacks a predefined format. It includes text documents, social media posts, images, videos, and audio files. The majority of data generated today is unstructured. AI, particularly through machine learning and natural language processing, is crucial for analyzing unstructured data and extracting meaningful information from it.
The Role of Data Analysis in AI Solutions
The ability to analyze data effectively is crucial in the development of AI systems. This process involves several steps, each of which contributes to the eventual success of the AI model.
Data Collection
Data collection is the first step in any AI data analysis process. It involves gathering relevant data from various sources to serve as the foundation for AI algorithms. Data can be collected through different methods, such as sensors, databases, surveys, or scraping the web. However, collecting vast amounts of data alone is not enough. Ensuring the relevance and accuracy of the data is equally important. The collected data must represent the problem space accurately to avoid biases or gaps that may affect the AI model’s performance.
Data Cleaning and Preprocessing
Raw data is often incomplete, noisy, or inconsistent. Data cleaning is the process of identifying and correcting errors or inconsistencies in the data to ensure high quality. This step involves removing duplicate records, handling missing values, and dealing with outliers that can skew the results. After cleaning, preprocessing transforms the data into a suitable format for analysis, which might involve normalization, categorization, or encoding.
For example, if AI is being applied to analyze customer feedback, data cleaning may involve removing irrelevant comments, normalizing sentiment scores, or encoding textual data into numerical form. Clean and well-preprocessed data lays the groundwork for more accurate and reliable AI predictions.
Data Exploration and Visualization
Once the data has been cleaned, it’s time for data exploration. Data exploration allows analysts and data scientists to delve deeper into the data to understand its structure, patterns, and underlying relationships. This phase involves using statistical methods, querying techniques, and visualization tools to uncover hidden insights. Data visualization tools, such as charts and graphs, provide a clear picture of trends, distributions, and outliers in the data.
This exploratory step helps in understanding the key variables influencing the outcome and how different factors interact with each other. It also aids in identifying which features will be most beneficial for AI model training, enhancing the model’s accuracy and effectiveness.
Feature Engineering
Feature engineering is the process of selecting, modifying, or creating new variables (features) from raw data to improve the performance of AI models. Not all features in a dataset will contribute to the predictive power of an AI solution. In fact, irrelevant or redundant features can hinder the performance of the model, leading to slower computations and less accurate results.
By carefully selecting and engineering the right set of features, data scientists can significantly improve the performance of AI algorithms. Techniques such as dimensionality reduction or feature scaling can be applied to optimize the data for faster and more accurate AI model predictions.
Model Selection and Training
After the data has been thoroughly analyzed, cleaned, and features have been engineered, the next step involves selecting the appropriate AI model. This is where machine learning algorithms come into play. Various algorithms are used depending on the nature of the problem and the type of data being analyzed. For example, regression models are used for predicting continuous outcomes, while classification models are applied for categorical predictions.
Once the model is selected, it undergoes a training process using the dataset. During this phase, the AI model learns patterns, trends, and relationships within the data. Training requires a significant amount of computational power, and it’s essential to ensure that the data fed into the model is balanced and unbiased to avoid overfitting or underfitting.
Leveraging AI Data Analysis for Business Solutions
AI data analysis is not just a technological process; it’s a business enabler. Many industries, including healthcare, finance, retail, and manufacturing, have adopted AI solutions to drive efficiency, reduce costs, and create better customer experiences.
Personalized Customer Experiences
In the world of e-commerce and digital marketing, AI data analysis is used to personalize customer experiences. By analyzing browsing history, purchase patterns, and demographic data, AI algorithms can recommend products, offer personalized discounts, or predict future buying behavior. This level of personalization leads to increased customer satisfaction and higher conversion rates.
Predictive Analytics
Predictive analytics is another valuable application of AI-driven data analysis. In industries such as finance and healthcare, AI models can analyze historical data to forecast future trends and outcomes. For instance, banks use AI to predict loan defaults, while healthcare providers use it to predict patient readmission rates. These predictive insights allow organizations to make proactive decisions, mitigating risks and improving operational efficiency.
Process Automation
AI solutions that rely on data analysis can also help businesses automate routine tasks and streamline workflows. In manufacturing, AI-powered robots can analyze production data to optimize assembly lines, while in customer service, chatbots use natural language processing to handle customer inquiries efficiently. This level of automation leads to significant cost savings and frees up human workers to focus on more complex and creative tasks.
Challenges in AI Data Analysis
Despite its vast potential, AI data analysis comes with its own set of challenges.
Data Privacy and Security
With the increased reliance on data, privacy concerns have become a major issue. Organizations need to ensure that they are complying with regulations such as the General Data Protection Regulation (GDPR) when collecting, storing, and processing customer data. Breaches in data privacy can result in legal penalties and loss of trust from customers.
Data Quality and Availability
Another challenge lies in the quality and availability of data. If the data used for AI model training is incomplete, outdated, or biased, the AI solution will be unreliable. Data collection efforts must be thorough, and companies need to invest in data management practices to ensure their data remains relevant and accurate.
Computational Costs
Analyzing large datasets and training AI models require considerable computational resources. For small businesses, the cost of acquiring the necessary infrastructure can be prohibitive. Cloud-based AI platforms, however, offer an alternative, providing scalable resources without the need for upfront hardware investments.
Conclusion
AI data analysis is the driving force behind many of today’s advanced AI solutions. By leveraging data effectively, organizations can gain valuable insights, predict future trends, and automate processes that were previously manual and time-consuming. While challenges such as data privacy and quality remain, the potential benefits of AI data analysis far outweigh the hurdles. As industries continue to embrace AI, the role of data analysis will only become more critical in unlocking the full potential of AI-driven innovations.
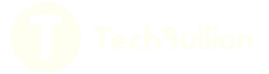