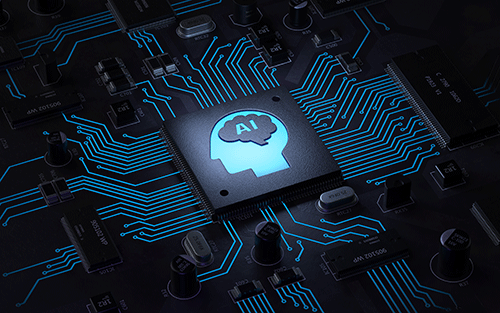
Summary
The companies winning the future of industry will be those who build a foundation for AI decision support now.

Artificial intelligence (AI) is one of the most popular topics in media right now, and for good reason. With the rise of ChatGPT specifically, and generative AI in particular, the world is beginning to see the power of these emerging intelligent tools. Organizations are already imagining a second Industrial Revolution built around an AI-assisted workforce, empowering personnel to do more with less, while improving the safety, sustainability, and performance of industrial operations worldwide.
A catalyst for new technology
The power industry—like many others—is experiencing a confluence of changes building the perfect environment for the rise of AI. With each passing year, more and more seasoned professionals are leaving the industrial workforce to be replaced by less experienced workers. When they leave, these skilled operators take with them decades of knowledge of how to optimally run plants. The loss of that knowledge can be devastating—particularly when the plant faces abnormal conditions for which automation was never designed.
Simultaneously, organizations are increasingly collapsing the operation of multiple plants into a single, remote operations center to make the most of limited resources. In such cases, small groups of operators are almost always monitoring a much broader spectrum of plants. They tend to need deeper knowledge to be successful, which can be a daunting task for newer personnel who are not familiar with the normal versus abnormal operations of each plant.
Increasing AI support
As organizations navigate consolidated operations and a less experienced workforce, they are increasingly discovering a need for additional automation. For years, operations teams have built failure mode and effects analysis charts and other first principles information. This data was coded into advanced automation using Bayesian statistics to help systems identify the root cause of problems, and then automatically intervene where possible. This complex programming created the foundation of adaptive and self-optimizing plants that has emerged in the last couple of decades.
However, it is difficult, if not impossible, to build explicit logic and rules to handle all the possible abnormal conditions that can show up in a plant. Many of a plant’s most challenging aberrations are those that are entirely unexpected—often caused by a wide array of factors that seem minimal on their own but together create turmoil.
A plant’s engineers cannot plan for every possible combination of process failures, but those limitations fall away as AI gains a stronger foothold in industrial automation. AI tools can take vast amounts of multivariate data and compare it and trend different process variables and states to gain a higher-level understanding of the process itself. Most importantly, AI solutions can perform this comparison and calculation much faster than a human engineer or operator.
The details are in the data
Data, specifically high-quality data, is critical to the accuracy and effectiveness of guidance provided by AI tools. There are two important ways AI engines will collect process data to work in tandem with personnel to increase operational excellence. The simplest method to train AI tools is through historical data. Today’s plants typically have massive amounts of historical operations and maintenance data, and a well-designed AI system can consume that data in very little time. Once an AI engine has consumed a plant’s historical data, it can build a picture of baseline operations and develop a conceptual framework for how and why processes diverge from specification.
In more advanced operations, teams will also likely couple AI tools with real-time digital twin simulations that continually train the AI models to recognize when normal and abnormal conditions occur. Based on the wealth of knowledge AI systems gain from digital twin training and forecasting, they will be able to work side-by-side with operators of any experience level to help diagnose problems, and then suggest or automatically implement the control actions necessary to get processes back on track—or at least maintain safe operating conditions.
Real-world impact
The potential value of AI assistance resonates strongly, for example, in one industrial sector currently undergoing rapid change: power generation. As renewables secure an ever-increasing market share of the power industry, the dynamics of how operators interact with the grid are changing quickly. Moreover, power generation is an industry ripe with transition to remote operations, where small teams of operators are commonly monitoring and managing fossil, solar, wind, battery, and other generation types across many facilities from a single remote location.
With renewable generation, changes happen quickly. When the sun stops shining or the wind stops blowing, the ripple effects quickly impact the dynamics of power systems. AI tools have the potential to identify, or even predict, emerging problems very quickly, and then notify human operators through their control interface to guide them through the steps to manage change in real-time, or even in advance. Additionally, AI models can include predictive data around weather and other variables to provide prognostic response, without clogging up an operator interface with confusing external data (Figure 1).
But power generation is only a single example where AI would provide a real impact. The value of AI extends far beyond a single industry. Nearly every modern plant needs to increase sustainability and performance to compete in a global marketplace, all while increasing uptime. As external factors increase in complexity, AI tools will be critical to closing the performance gap.
Preparing for the future
The next Industrial Revolution, precipitated by a rise in AI technologies, may be many years away. However, as that future draws near, gradually emerging AI applications have the potential to be game changers, unlike any other technology in the careers of current plant personnel. The companies not prepared to adopt those technologies are likely to fall behind.
To address that and other issues, forward-thinking organizations are assessing their current infrastructure and taking gradual steps to modernize their systems. The powerful AI solutions of the future will require the data democratization made possible through a Boundless Automation vision—enabled by technologies that move data seamlessly from the intelligent field, through the edge, and into the cloud. Starting to incorporate that vision today will lay the foundation necessary to drive the performance and sustainability that will be demanded in tomorrow’s operations.
All figures courtesy of Emerson
About The Author
Rick Kephart has more than 30 years of automation experience in the power and water/wastewater industries. Over his career he has become an expert in control systems and theory, embedded systems, and real-time systems. Rick currently serves as the vice president of technology for Emerson’s power and water solutions business. In this position, Rick leads the global power and ewater technology organization, where he is responsible for shaping technology architecture, strategy, and direction for the Ovation platform, and the entire power generation and water system stacks. He holds a B.S. in electrical engineering from Penn State University and an M.S. in electrical engineering from the University of Pittsburgh.
Did you enjoy this great article?
Check out our free e-newsletters to read more great articles..