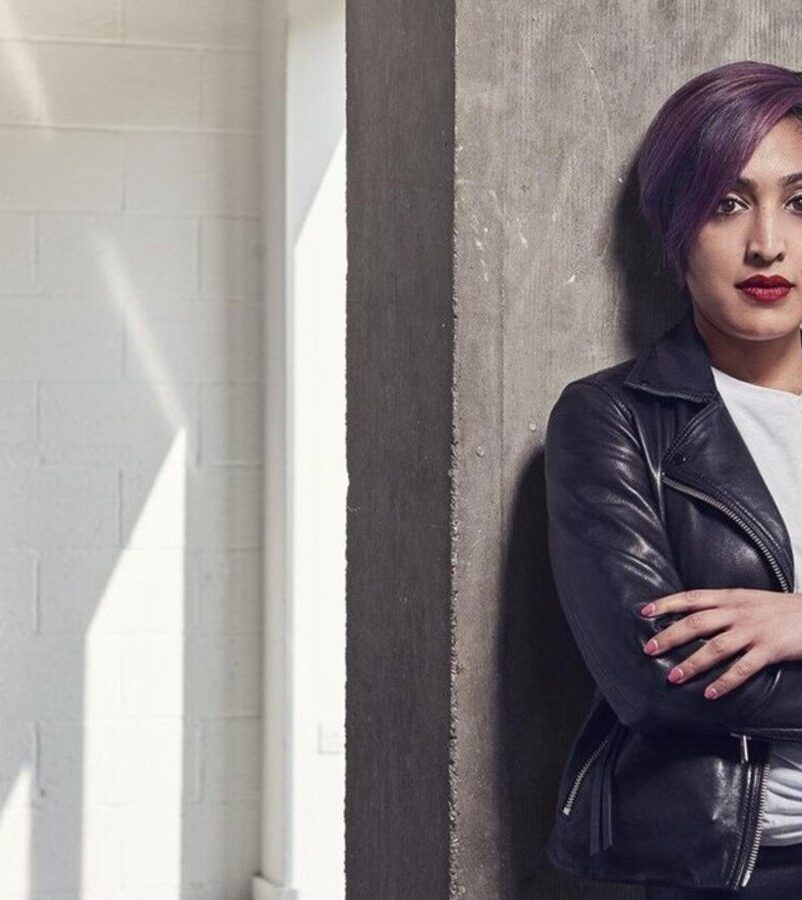
Dr. Rumman Chowdhury has built solutions in the field of applied algorithmic ethics since 2017. She is the CEO and co-founder of Humane Intelligence, a nonprofit dedicated to algorithmic access and transparency, and was recently named one of Time Magazine’s 100 Most Influential People in AI 2023. Previously, she was the Director of the Machine Learning Ethics, Transparency, and Accountability team at Twitter.
Artificial intelligence’s transformational possibility is currently the focus of conversations at everything from kitchen tables to UN Summits. What can be built today with AI to solve one of society’s big challenges, and how can we drive attention and investment towards it?
Hand in hand with investment in technological innovation needs to be investment in the forms of AI systems that can protect humans from the augmentation of algorithmic bias. This might include new techniques for adversarial AI models that identify misinformation, toxic speech, or hateful content; this could mean more investment in proactive methods of illegal and malicious deepfake identification, and more.
More on:
Driving investment to this is simple: for every funding ask to develop some new AI capability must be equal investment in the research and development of systems to mitigate the inevitable harms that will follow.
The data underlying large language models raises fundamental questions about accuracy and bias, and whether these models should be accessible, auditable, or transparent. Is it possible to establish meaningful accountability or transparency for LLMs, and if so, what are effective means of achieving that?
Yes, but defining transparency and accountability has been the trickiest part. A new resource from Stanford’s Center for Research on Foundation Models (CRFM) illustrates how complex the question is. The Center recently published a new index on the transparency of foundational AI models, which scores the developers of foundational models (companies such as Google, OpenAI, and Anthropic) against one hundred different indicators designed to characterize transparency. This includes everything from transparency regarding what went into building a model, to the model’s capabilities and risks, to how it is being used. In other words, clarifying what meaningful transparency looks like is a huge question, and one that will continue to evolve. In addition, accountability is tricky as well. We want harms to be identified and addressed proactively, but it is hard to consider a method of accountability that is not reactive.
However, in a soon-to-be-published study that I’m conducting, I find that, broadly speaking, most model evaluators (defined very broadly) want the same things—they want secure access to an application programming interface, they want datasets they can use to test models, they want an idea of how the model is used and how it participates in an algorithmic system, and they want the ability to create their own test metrics. Interestingly not a single interviewee asked for model data or code directly, which is concerning as this is often a controversial touchpoint between regulators, policymakers, and companies.
Artificial intelligence is a multi-use technology, but that does not necessarily mean it should be used as a general purpose technology. What potential uses of AI most concern you and can those be constrained or prevented?
Any unmediated use that directly makes a decision on the quality of life of a human being. By “unmediated” I mean, without meaningful human input and ability to make an informed decision regarding the model. This applies to a massively broad range of uses for AI systems.
The market of powerful AI tools is growing exponentially, as is easy, public access to those tools. Although calls for AI governance are increasing, governance will struggle to keep pace with AI’s market and technological evolution. What elements of AI governance are most critical to achieve in the immediate terms, and which elements are the most possible to achieve in the immediate term?
I do not think what we need is regulation that moves at the pace of every new innovation, but regulatory institutions and systems that are flexible to the creation of new algorithmic capabilities. What we lack today in Responsible AI are legitimate, empowered institutions that have clear guidelines, remits, and subject matter expertise.
Mission critical are: transparency and accountability (see above), clear definitions of algorithmic auditing, and legal protections for third party assessors and ethical hackers.
More on:
Large digital platforms will be a key vector for disseminating AI-generated content. How can existing standards and norms in platform governance be leveraged to mitigate the spread of harmful AI-generated content, and how should they be expanded to address that threat?
Generative AI will supercharge the dissemination of deepfake content for malicious use. While insufficient, we can learn from how platforms have used narrow AI and ML alongside human decisioning to address issues of toxicity, radicalization, online bullying, online gender-based violence, and more. These systems, policies, and approaches need to be significantly invested in and improved.
Is there anything else you’d like to address about AI development or governance?
The missing part of the story is public feedback. Today there is a broken feedback loop between the public, government and companies. It’s important to invest in methods of structured public feedback—everything ranging from expert and broad-based red teaming, bias bounties, and more—to identify and mitigate AI harms.
In an ongoing series of interviews, we ask AI governance experts the same five questions, and then allow them to close by highlighting what other question or issue they would like to address. We’ll be highlighting a range of different experts throughout the year. This article is the second in an ongoing series; to read our first interview, with Google’s Kent Walker, click here.