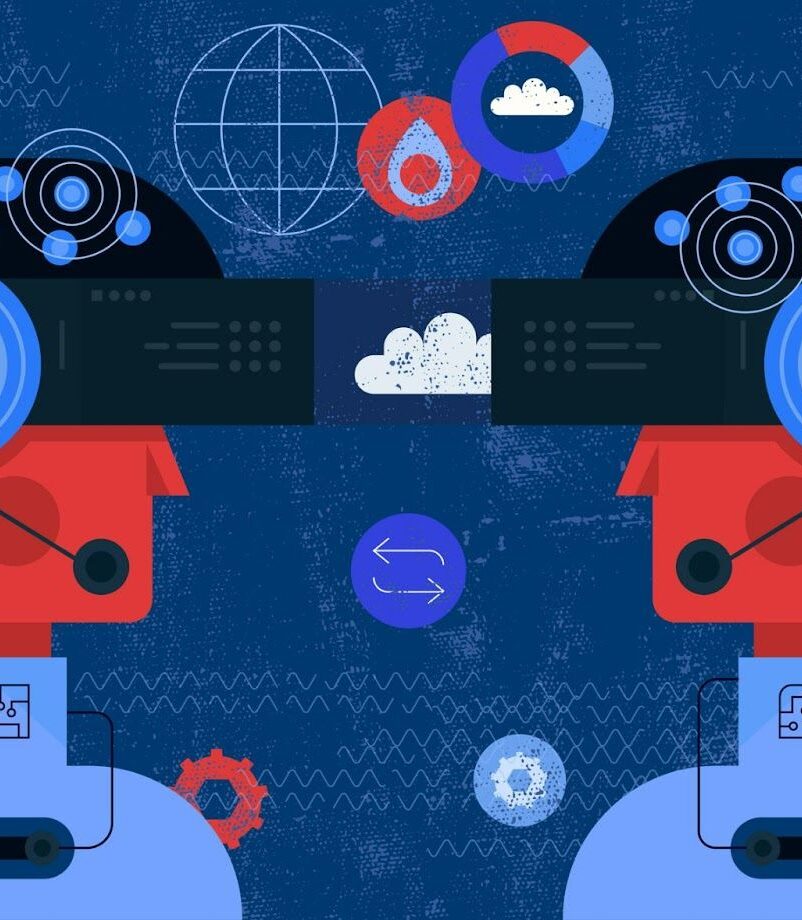
The last two years have been some of the most exciting and highly anticipated in Automatic Speech Recognition’s (ASR’s) long and rich history, as we saw multiple enterprise-level fully neural network-based ASR models go to market (e.g. Alexa, Rev, AssemblyAI, ASAPP, etc). The accelerated success of ASR deployments is due to many factors, including the growing ecosystem of freely available toolkits, more open source datasets, and a growing interest on the part of engineers and researchers in the ASR problem. This confluence of forces has produced an amazing momentum shift in commercial ASR. We truly are at the onset of big changes in the ASR field and of massive adoption of the technology.
These developments are not only improving existing uses of the technology, such as Siri’s and Alexa’s accuracies, but they are also expanding the market ASR technology serves. For example, as ASR gets better with very noisy environments, it can be used effectively in police body cams, to automatically record and transcribe interactions. Keeping a record of important interactions, and perhaps identifying interactions before they become dangerous, could save lives. We are seeing more companies offering automated captions to live videos, making live content accessible to more people. These new use-cases and customers are pushing the requirements for ASR, which is accelerating research.
What’s Next for ASR?
“By 2030, speech recognition will feature truly multilingual models, rich standardized output objects, and be available to all and at scale. Humans and machines will collaborate seamlessly, allowing machines to learn new words and speech styles organically. Finally, speech recognition will engender the principles of responsible AI, and operate without bias.”
Source: Hannun, Awni, “Speech Recognition is not Solved”.
In 2016, 6 years ago already, Microsoft Research published an article announcing they had reached human performance (as measured using Word Error Rate, WER) on a 25-year old data set called Switchboard. As Zelasko et al. have pointed out, such optimistic results do not hold up on data reflecting natural human speech spoken by a diverse population.
Nevertheless, ASR accuracy has continued to improve, reaching human parity on more datasets and in more use cases. On top of continuing to push the limits of accuracy for English systems, and, in doing so, redefining how we measure accuracy as we approach human-level accuracy, we foresee five important areas where research and commercial systems will evolve in the next ten years.
Truly Multilingual ASR Models
“In the next decade, we see fully multilingual models being deployed in production environments, enabling developers to build applications that can understand anybody in any language, truly unlocking the power of speech recognition to the world at large.”
Source: Conneau, Alexis, et al. “Unsupervised cross-lingual representation learning for speech recognition.” arXiv preprint arXiv:2006.13979 (2020).
Today’s commercially available ASR models are primarily trained using English-language data sets and consequently exhibit higher accuracy for English-language input. Due to data availability and market demands, academia and industry have focused on English for a very long time. Accuracy for commercially popular languages like French, Spanish, Portuguese, and German is also reasonable, but there is clearly a long tail of languages for which limited training data exists and ASR output quality is correspondingly lower.
Furthermore, most commercial systems are monolingual, which doesn’t accommodate the multilingual scenarios characteristic of many societies. Multilinguality can take the form of back-to-back languages, for example in a bilingual country’s media programming. Amazon recently introduced a product integrating language identification (LID) and ASR that makes big strides toward handling this.In contrast, translanguaging (also known as code switching) involves individuals employing a linguistic system that incorporates both words and grammar from two languages potentially within the same sentence. This is an area where the research community continues to make interesting progress.
Just as the field of Natural Language Processing has taken up multilingual approaches, we see the world of ASR doing the same in the next decade. As we learn how to take advantage of emerging end-to-end techniques, we will be able to train massively multilingual models that can exploit transfer learning between multiple languages. A good example of this is Facebook’s XLS-R: in one demo you can speak any of 21 languages without specifying which and it will translate to English. These smarter ASR systems, by understanding and applying similarities between languages, will enable high-quality ASR availability for both low-resource languages and mixed-language use cases, and they will do so at a commercial quality-level.
Rich Standardized Output Objects
“In the next decade, we believe commercial ASR systems will return a much richer transcript object that will contain much more than simple words. Further, we foresee this richer output will be agreed upon by a standards organization such as W3C, so that all APIs return similarly constructed outputs. This will further unlock the potential of voice applications for everyone in the world.”
While there is a long tradition of exploration into “rich transcription”, originally by NIST, there has not been much effort on standardized and extensible formats for incorporating it into ASR output. The notion of rich transcription originally involved capitalization, punctuation and diarization, but has expanded somewhat into speaker roles and a range of non-linguistic human speech events. Anticipated and desired innovations include the ability to transcribe potentially simultaneous and overlapping speech from different speakers, emotions and other paralinguistic characterizations, and a range of non-linguistic and even non-human speech scenes and events. It would also be possible to include stylistic or language variety-based information. Tanaka et al. describe a scenario where a user might want to choose among transcription options at different levels of richness, and clearly the amount and nature of the additional information we foresee would be specifiable, depending on the downstream application.
Traditional ASR systems are capable of generating a lattice of multiple hypotheses in their journey to identify spoken words, and these have proved useful in human-assisted transcription, spoken dialog systems, and information retrieval. Clearly, including n-best information along with confidence in a rich output format will encourage more users to exploit it, improving user experiences. While no standard currently exists for structuring or storing the additional information currently generated or possible to generate during the speech decoding process, one promising step in this direction is CallMiner’s Open Voice Transcription Standard (OVTS) which makes it easier for enterprises to explore and use multiple ASR vendors.
We predict that in future, ASR systems will produce richer output in a standard format that will enable more powerful downstream applications. For example, an ASR system might return the whole lattice of possibilities as its output, and an application could use this additional data for intelligent auto-complete when editing the transcript. Similarly, ASR transcripts that include additional metadata (such as detected regional dialects, accents, environmental noise and/or emotions) could enable more powerful search applications.
ASR for All and At Scale
“In this decade, ASR at scale, meaning private, affordable, reliable, and fast, will become the norm for everyone. These systems will enable searching through videos, indexing all media content we participate in, and making every video accessible for hearing-impaired consumers around the world. ASR will be the key to turn every audio and video into something accessible and actionable.”
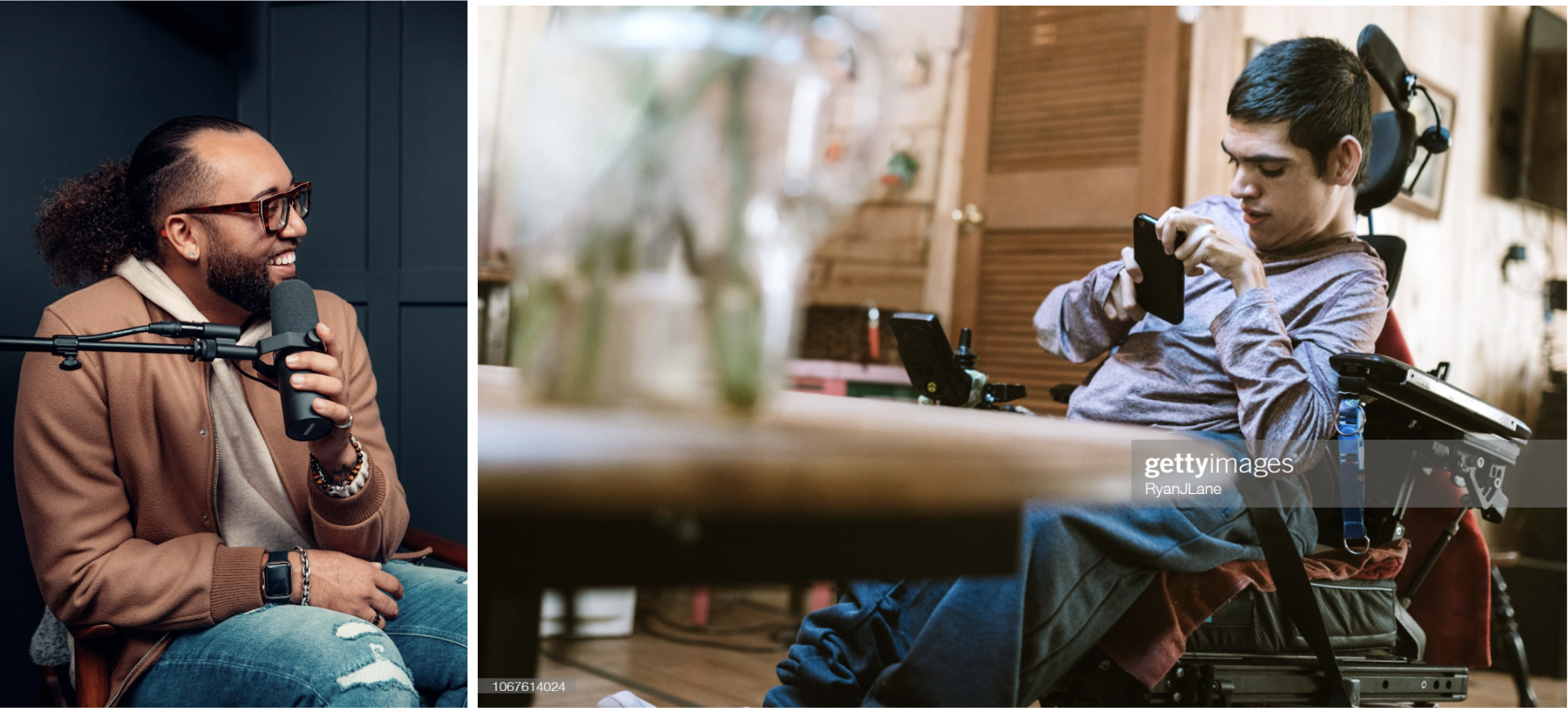
We are all consuming (and participating in) massive amounts of content: podcasts, social media streams, online videos, real-time group chats, Zoom meetings and many more. However, very little of this content is actually transcribed. Today, content transcription is already one of the largest markets for ASR APIs and is set to grow exponentially in the next decade, especially given how accurate and affordable they are becoming. Having said this, ASR transcription is currently used only in select applications (broadcast videos, some meetings, some podcasts, etc.). As a result, this media content is not accessible for many people and it is extremely difficult to find information after a broadcast or event is over.
In the future, this will change. As Matt Thompson predicted in 2010, at some point ASR will be so cheap and widespread that we will experience what he called “The Speakularity”. We will expect almost all audio and video content to be transcribed and become immediately accessible, storable and searchable at scale. And it won’t stop there. We will want this content to be actionable. We will want additional context for each piece of content we consume or participate in, such as auto-generated insights from podcasts or meetings, or automatic summaries of key moments in videos… and we will expect our NLP systems to produce these for us as a matter of routine.
Human-Machine Collaboration
“By the end of this decade, we predict we will have constantly evolving ASR systems, much like a living organism, that continuously learn with the help of humans or by self-supervision. These systems will learn from diverse sources in the real world, in a live fashion rather than in an asynchronous fashion, understanding new words and language varieties, self-debugging and automatically monitoring for different usages.”
As ASR becomes more mainstream and covers an ever-increasing number of use cases, human-machine collaboration is set to play a key role. ASR model training is a good example of this. Today, open source data sets and pre-trained models have reduced the barriers to entry for ASR vendors. However, the training process is still fairly simplistic: collect data, annotate the data, train a model, evaluate results, repeat, to iteratively improve the model. This process is slow and, in many cases, error-prone due to difficulties in tuning or insufficient data. Garnerin et al. have observed that a lack of metadata and inconsistency in representations across corpora have made it harder to provide equivalent accuracy to all communities in ASR performance; this is something that Reid & Walker are also trying to address with the development of metadata standards.
In the future, humans will play an increasingly important role in accelerating machine learning through intelligent and efficient supervision of ASR training. The human-in-the-loop approach places human reviewers inside the machine learning/feedback cycle, allowing for ongoing review and tuning of model results. This results in faster and more efficient machine learning leading to higher-quality outputs. Earlier this year we discussed how ASR improvements have enabled Rev’s human transcriptionists (known as “Revvers”), who post-edit an ASR draft, to be even more productive. Revver transcriptions feed right into improved ASR models, creating a virtuous cycle.
One area where human language experts are still indispensable in ASR is inverse text normalization (ITN), where recognized strings of words like “five dollars” are converted to expected written forms like “$5”. Pusateri et al. describe a hybrid approach using “both handcrafted grammars and a statistical model”; Zhang et al. continue along these lines by constraining an RNN with human-crafted FSTs.
Responsible ASR
“ASR systems of the future, like all AI systems, will adhere to stricter responsible AI principles, so that systems treat all people equally, are more explainable, accountable for their decisions, and respect the privacy of users and their data.”
ASR systems of the future will be expected to adhere to the four principles of responsible AI: fairness, explainability, accountability and respect for privacy.
-
Fairness: Fair ASR systems will recognize speech regardless of the speaker’s background, socio-economic status or other traits. It is important to note that building such systems requires recognizing and reducing bias in our models and training data. Fortunately, governments, non-governmental organizations and businesses have begun creating infrastructure for identifying and alleviating bias.
-
Explainability: ASR systems will no longer be “black boxes”: they will provide, on request, explanations of how data is collected and analyzed and on a model’s performance and outputs. This additional transparency will result in better human oversight of model training and performance. Along with Gerlings et al., we view explainability with respect to a constellation of stakeholders, including researchers, developers, customers and in Rev’s case, transcriptionists. Researchers may want to know why erroneous text was output so they can mitigate the problem, while transcriptionists may want some evidence why ASR thought that’s what was said, to help with their assessment of its validity, particularly in noisy cases where ASR may “hear” better than people do. Weitz et al. have taken important initial steps towards end-user explanations in the context of audio keyword spotting. Laguarta & Subirana have incorporated clinician-directed explanations into a speech biomarker system for Alzheimer’s detection.
-
Respect for privacy: “Voice” is considered “personal data” under various US and international laws, and the collection and processing of voice recordings is therefore subject to stringent personal privacy protections. At Rev, we already offer data security and control features, and future ASR systems will go even further to respect both the privacy of user data and the privacy of the model. Most likely, in many cases, this will involve pushing ASR models to the edge (on devices or browsers). The Voice Privacy Challenge is motivating research in this area, and many jurisdictions, such as the EU, have begun adopting guidelines and legislation. The field of Privacy Preserving Machine Learning promises to facilitate emphasis on this critical aspect of our technology so that it can be widely embraced and trusted by the general public.
-
Accountability: ASR systems will be monitored to ensure that they adhere to the previous three principles. This in turn will require a commitment of resources and infrastructure to design and develop the necessary monitoring systems and take actions on their findings. Companies that deploy ASR systems will be expected to be accountable for their use of the technology and in making specific efforts to adhere to responsible ASR principles.
It’s worthwhile mentioning that as the designers, maintainers and consumers of ASR systems, humans will be responsible for implementing and enforcing these principles – yet another example of human-machine collaboration.
Conclusion
Many of these advances are already well under way, and we fully expect the next ten years to be quite an exciting ride for ASR and related technologies. At Rev, we’re excited to make progress in all these topics, starting with the release of our v2 model surpassing previous state of the art by 30% relative based on internal and external test suites. Those interested in watching these trends take form, and catching up on the incredible advances of our sister technologies like text-to-speech (TTS) and speaker recognition, are encouraged to attend the field’s upcoming technical conferences, Interspeech, ICASSP and SLT. For those with a more linguistic bent, COLING and ICPhS are recommended, and for a business-oriented overview, Voice Summit.
What do you think?
We’d love to hear your predictions as well.
Citation
For attribution in academic contexts or books, please cite this work as
Migüel Jetté and Corey Miller, “The Future of Speech Recognition: Where will we be in 2030?”, The Gradient, 2022.
BibTeX citation:
@article{miller2021futureofowork,
author = {Jetté, Migüel and Miller, Corey},
title = {The Future of Speech Recognition: Where will we be in 2030?},
journal = {The Gradient},
year = {2022},
howpublished = {\url{https://thegradient.pub/the-future-of-speech-recognition/} },
}