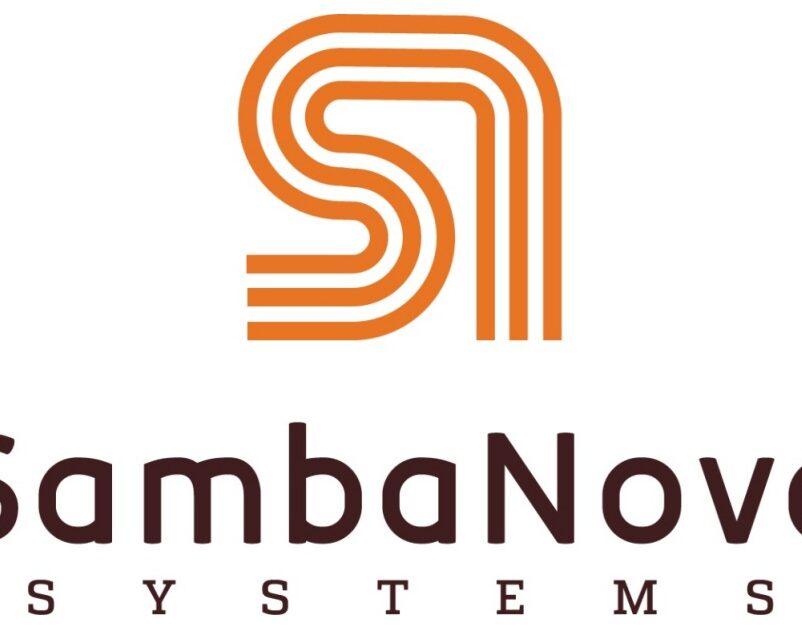
There is a lot of hype and activity around generative AI models, especially in the short time since ChatGPT first launched.
ChatGPT — and the GPT-3 large language model (LLM) it is based on — have been trained on public data, which serves as a great foundation for consumer applications, but lacks the customization, privacy and security that an enterprise requires. That’s where Rodrigo Liang, cofounder and CEO of SambaNova Systems, is looking to make a difference with the launch today of his company’s SambaNova Suite, which aims to help enterprises build and deploy customized generative AI models.
>>Follow VentureBeat’s ongoing generative AI coverage<<
SambaNova got its start in 2017 focused largely on hardware for AI, raising a staggering $676 million in April 2021 to support its efforts. In recent years, the company has expanded beyond its initial focus on hardware to also build out support for both machine learning–training and inference on different models, with its dataflow-as-a-service offering. The new SambaNova Suite expands on the dataflow service with a collection of capabilities that enables organizations to customize both open-source and proprietary generative AI models to meet their specific requirements.
“The focus of SambaNova is how to bring generative AI capabilities more to the enterprise,” Liang told VentureBeat. “There are certain things that you need for enterprises in order to be able to be successful, and we’re doing that today for them.”
Nvidia isn’t the only AI hardware vendor for generative AI
There is a growing list of vendors building on top of generative AI models to help enable enterprise use cases.
Content creation is a particularly vibrant field of enterprise generative AI customization. Jasper AI, for example, recently announced its Jasper for Business offering designed to help customize AI for a specific business. Typeface emerged from stealth on Monday with its enterprise content creation platform for generative AI, alongside $65 million in funding. Quantive last week announced its foray into generative AI, helping organizations with their business strategy.
SambaNova’s key differentiator from others in the generative AI space is that it has its own hardware to help optimize enterprise use cases. Rather than just relying on Nvidia GPUs like many in the industry, SambaNova has developed its own custom silicon that is optimized for both machine learning–training and inference.
“What we’ve done is … take the AI software stack that people really want to use with PyTorch, TensorFlow and complex models like GPT, and we bring them down all the way to the silicon,” Liang said. “We have silicon that’s actually been custom built for running these large models for the enterprise, versus the other way around.”
The team behind SambaNova, including Liang, has a background in building microprocessors for Sun Microsystems and Oracle. Liang said that SambaNova has created a microprocessor for AI that is extremely power efficient and performative in order to run these enterprise generative AI applications.
Liang emphasized that the custom silicon also enables a continuous training and inference capability, such that the data that feeds the generative AI models can be kept up to date.
“In business, you need real-time information and so you do not want your models to actually lag behind,” he said.
Privacy, responsible AI and the enterprise
With the new SambaNova Suite, Liang said that his company is looking to solve the primary challenges that enterprises have with generative AI. Among those challenges are customization for a company’s specific data, as well the ability to limit bias and provide responsible and explainable AI.
With its platform, SambaNova enables an organization to run customized training in a private environment on any data the organization has, including unstructured data that might be found in a company’s Slack discussion channels. Going a step further, Liang said the platform provides transparency to organizations for how a given AI model actually works.
“SambaNova has been built around being able to give you exposure of exactly how the model arrived at a certain conclusion,” Liang said. “We store all the processes around how we train and fine-tune the model, so that when an auditor comes or somebody wants to check for bias or why something happened a certain way, you can actually work through the flow and verify that your results were done properly.”
VentureBeat’s mission is to be a digital town square for technical decision-makers to gain knowledge about transformative enterprise technology and transact. Discover our Briefings.